Priyadarshini Panda
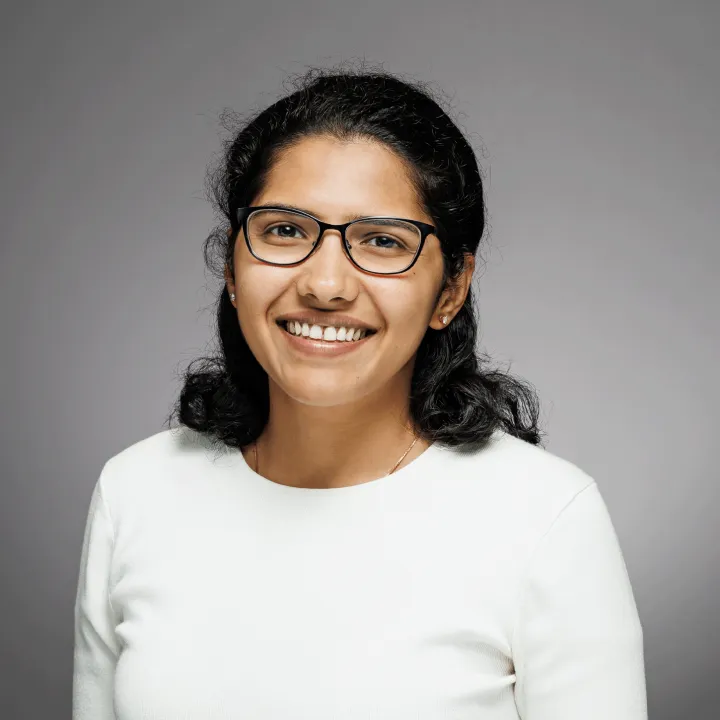
Assistant Professor of Electrical & Computer Engineering
Phone:
(203) 432-1203
Room / Office:
Dunham 517
Office Address:
10 Hillhouse Avenue
New Haven, CT 06511
Mailing Address:
P.O. Box 208267
New Haven, CT 06520
About Priyadarshini Panda
Degrees
- Ph.D., Purdue University
- M.Sc., B.I.T.S. Pilani
- B.E., B.I.T.S. Pilani
Perspectives
Priya's research interests lie in Neuromorphic Computing: spanning energy-efficient design methodologies for deep learning networks, novel supervised/unsupervised learning algorithms for spiking neural networks and developing neural architectures for new computing scenarios (such as lifelong learning, generative models, stochastic networks, adversarial attacks etc.).
Her goal is to empower energy-aware and energy-efficient machine intelligence through algorithm-hardware co-design while being secure to adversarial scenarios and catering to the resource constraints of Internet of Things (IoT) devices.
Selected Publications
A comprehensive list of publications is available here.
Selected Publications (Journal):
- Kaushik Roy, Akhilesh Jaiswal, and Priyadarshini Panda. Towards Spike-based Machine Intelligence with Neuromorphic Computing. To appear in Nature (2019). An online tutorial on the paper encompassing the perspectives on neuromorphic computing field is available on "https://www.youtube.com/watch?v=HnxkQvPcdXs".
- Fan Zuo*, Priyadarshini Panda*, Michele Kotiuga, Jiarui Li, Mingu Kang, Claudio Mazzoli, Hua Zhou et al. Habituation based synaptic plasticity and organismic learning in a quantum perovskite. Nature communications 8, no. 1 (2017): 240 (*Equal author contributions).
- Priyadarshini Panda, Swagath Venkataramani, Abronil Sengupta, Anand Raghunathan, and Kaushik Roy. Energy-efficient object detection using semantic decomposition. IEEE Transactions on Very Large Scale Integration (VLSI) Systems, doi:10.1109/TVLSI.2017.2707077, 25(9):2673–2677, Sept 2017.
- Priyadarshini Panda, Indranil Chakraborty, and Kaushik Roy. Discretization based Solutions for Secure Machine Learning against Adversarial Attacks. IEEE Access (2019).
- Abhronil Sengupta, Priyadarshini Panda, Parami Wijesinghe, Yusung Kim, and Kaushik Roy. Magnetic tunnel junction mimics stochastic cortical spiking neurons. Scientific reports (2016): 30039.
- Deboleena Roy, Priyadarshini Panda, and Kaushik Roy. Tree-cnn: A hierarchical deep convolutional neural network for incremental learning. arXiv preprint arXiv:1802.05800, 2018, Accepted in Neural Networks (Elsevier), 2019.
- Chankyu Lee, Priyadarshini Panda, Gopalakrishnan Srinivasan, and Kaushik Roy. Training deep spiking convolutional neural networks with stdp-based unsupervised pre-training followed by supervised finetuning. Frontiers in Neuroscience, 12:435, 2018.
Selected Publications (Conference):
- Priyadarshini Panda, Abhronil Sengupta, and Kaushik Roy. Conditional deep learning for energy-efficient and enhanced pattern recognition. In 2016 Design, Automation & Test in Europe Conference & Exhibition (DATE), pp. 475-480. IEEE, 2016.
- Priyadarshini Panda, and Kaushik Roy. Unsupervised regenerative learning of hierarchical features in spiking deep networks for object recognition. In 2016 International Joint Conference on Neural Networks (IJCNN), pp. 299-306. IEEE, 2016.
- Priyadarshini Panda and Kaushik Roy. Implicit generative modeling of random noise during training for adversarial robustness. arXiv preprint arXiv:1807.02188, In ICML 2019 - Workshop on Uncertainty and Robustness in Deep Learning.
- Priyadarshini Panda, Abhronil Sengupta, Syed Shakib Sarwar, Gopalakrishnan Srinivasan, Swagath Venkataramani, Anand Raghunathan, and Kaushik Roy. Cross-layer approximations for neuromorphic computing: From devices to circuits and systems. In 2016 53nd ACM/EDAC/IEEE Design Automation Conference (DAC), pp. 1-6. IEEE, 2016.
- Aayush Ankit, Abhronil Sengupta, Priyadarshini Panda, and Kaushik Roy. Resparc: A reconfigurable and energy-efficient architecture with memristive crossbars for deep spiking neural networks. In Proceedings of the 54th Annual Design Automation Conference 2017, p. 27. ACM, 2017.